IT
How to Unlock the Power of AI in Enterprise Architecture
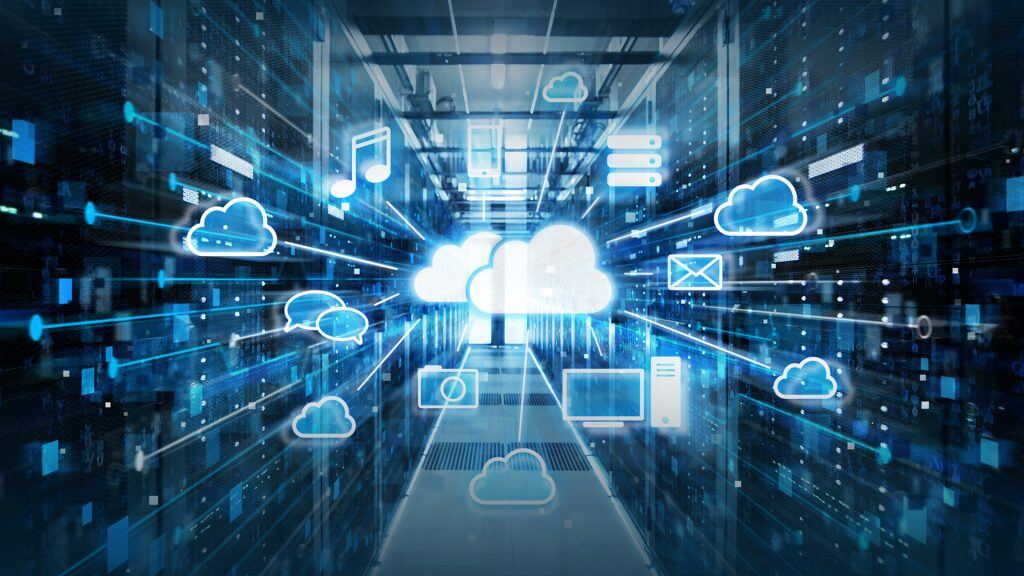
Be part of the biggest events of the season
Apr 10, 2025 | 6 min read
AI is transforming every facet of an organization’s operations, and enterprise architecture is no exception. AI has the potential to revolutionize the way enterprise architects drive business growth in their organizations. According to a PayScale study, today’s enterprise architects are expected to have strong AI and business intelligence competencies. In this article, we explore how AI can assist common enterprise architecture tasks, the role of enterprise architects in AI adoption, and how AI can be harnessed to create an agile enterprise architecture.
How AI Can Support Enterprise Architects
A report by Bizzdesign lists the following as top priorities to drive enterprise architecture forward:
- Improving communication about how enterprise architecture adds value to organizations (56%)
- Accelerating the development of enterprise architecture processes (50%)
- Delivering more strategic insights (41%)
- Getting more support from senior management (33%).
AI presents viable solutions to realize those priorities and boost the organizational impact of enterprise architecture. Here are areas where AI can be applied:
Getting enterprise architecture projects off the ground
The early stages of an enterprise architecture project are crucial. Employing AI techniques such as machine learning and neural graph networks can lay a strong foundation for an enterprise architecture program. Without AI, identifying areas for improvement and finding relevant insights can be time-consuming, resource-intensive, and prone to human error. For example, enterprise architects can utilize AI tools to quickly and efficiently analyze large volumes of data to pinpoint areas that require improvement, such as process bottlenecks or areas lacking automation. With a more precise understanding of these areas, enterprise architects can develop a more effective program tailored to their organization’s specific needs.
Enabling data-driven analysis and decision-making
AI can help enterprise architects make more proactive decisions by delivering solutions based on data-driven analysis, identifying patterns and trends more effectively, and enabling real-time, multi-source data analysis. For instance, for enterprise architects in the automotive industry, AI can use advanced natural language processing (NLP) to analyze unstructured data, such as vehicle specifications, internal communications, and maintenance logs, to identify key insights and trends. With this information, enterprise architects can make informed decisions about strategies based on the current state of the business, supply chain trends, and production efficiency.
Reducing enterprise architecture project failure rates
Factors contributing to the failure of enterprise architecture projects include inadequate leadership support, poor stakeholder communication, and a limited grasp of business requirements. However, difficulty managing business complexity is the biggest pitfall with enterprise architecture projects. AI tools can help enterprise architects manage this complexity by providing insights and identifying patterns from large volumes of data from different sources. Additionally, AI tools can proactively identify potential roadblocks that could hinder project success. It can swiftly flag risks and dependencies that might impact project timelines, budgets, or scope.
What are Enterprise Architecture Tools with AI Capabilities?
The enterprise architecture tool sector is booming, with revenues growing up to 30% annually (Gartner). Consequently, today’s enterprise architects have access to a wide range of AI-enabled enterprise architecture tools. It’s important to explore and pilot solutions to identify which tools fit organizational needs and budget the best. Here are examples of readily available tools on the market:
- Ardoq: Employs machine learning to automatically discover and map an organization’s architecture.
- LeanIX: Leverages machine learning to enhance data quality, provide recommendations for application rationalization, and enable predictive analysis.
- QualiWare X: Uses machine learning to automate data population, create architecture diagrams, and suggest improvements based on historical data and best practices.
- Bizzdesign Enterprise Studio: Employs NLP to understand and process text-based data, enabling users to capture, analyze, and categorize information from documents.
- Sparx Systems Enterprise Architect: Integrates NLP for natural language querying of architectural models, making it easier for users to access and understand complex architecture data.
- Mega Hopex: Incorporates NLP for semantic data extraction and analysis, as well as interaction with architecture data.
Driving Innovation with Generative AI
A good enterprise architect is both a tech-savvy analyst and a creative thinker. However, the increasing complexity of IT landscapes and the processes that come with it eat up most of their time, leaving little time and energy for strategic thinking.
This is where generative AI tools come in. These tools can become valuable assets that assist with time-consuming tasks such as creating reports, conducting research, analyzing data, summarizing complex documents, and debugging code. In addition, generative AI tools provide a quicker way to gain insights into market trends, customer behavior, and competition to inform strategic decisions.
William El Kaim, IT Architecture Director at Boston Consulting Group cites five key areas where generative AI can support enterprise architects:
- Reduce the time spent on low-value tasks and enhance collaboration between business and IT
- Make enterprise architecture and technology governance more efficient
- Use up-to-date IT landscape data to improve the quality of analysis
- Design compliant architectures with guided design and automated model generation
- Accelerate knowledge transfer, training, and skills gap closure
- As generative AI tools like ChatGPT become more advanced, they will no doubt become an essential resource for enterprise architects. As Capgemini Architecture Director, Pascal Espinouse explains:
“Whether it be assisting with architecture design, component selection, or technical documentation generation, ChatGPT has the potential to transform the way architects work. It can ultimately increase their productivity, efficiency, and effectiveness.”
The Role of Enterprise Architects in AI Adoption
As enterprise architects are in charge of aligning technology strategies with business objectives, they can have a significant influence on the adoption, implementation, and governance of AI. Here are several initiatives enterprise architects can take on to help businesses adopt AI tools effectively:
- Identify where AI can add value: This gives enterprise architects an opportunity to gain a holistic understanding of the organization’s goals, capabilities, and resources. With ongoing collaboration with top management, enterprise architects possess the insights to identify which areas can benefit the most from AI and develop an AI implementation roadmap from there.
- Assess technical feasibility and risks: Enterprise architects can leverage their analytical skills here and do a deep dive into the organization’s existing infrastructure, data availability, and compatibility with AI systems to ensure AI is implemented successfully and safely.
- Develop an AI governance framework: Enterprise architects have a chance to work together with fellow IT colleagues to define policies, guidelines, and standards for data privacy, security, and algorithmic transparency to ensure the ethical and responsible use of AI throughout the organization.
When it comes to generative AI, McKinsey recommends five key elements that need to be incorporated into the technology architecture:
- Context management and caching provide models with relevant information from enterprise data sources. Access to data allows the model to understand context and produce outputs. Caching also stores results to enable faster responses.
- Policy management to ensure controlled and customized access to confidential enterprise data assets.
- Model hub with trained, approved models that can be provisioned on demand, storing model checkpoints, weights, and parameters.
- Prompt library containing optimized instructions for the generative AI models, including prompt versioning as models are updated.
- MLOps platform with upgraded MLOps capabilities considering the complexity of generative AI models. MLOps pipelines need instrumentation to measure task-specific performance, such as measuring a model’s knowledge retrieval.
As AI technologies continue to evolve, enterprise architects have a unique opportunity to drive organizational growth and innovation. By understanding the potential of these technologies and their application in different business domains, enterprise architects can design intelligent systems, optimize processes, and foster a culture of innovation.